Welcome to the world of data-driven organizations where it is crucial to have a well governed repository to efficiently store and manage your valuable data. But with so many options available, finding the right approach can be overwhelming.
When it comes to architecting an analytics-supporting data repository, there are two main approaches to consider. The first is the traditional three-tier relational approach, known as an Analytical Data Mart (ADM). The second is the popular data lake approach.
Each approach comes with its own unique advantages and disadvantages, allowing you to tailor your data storage solution to meet the specific requirements of your organization. In some more advanced organizations ADM and data lakes may even complement each other rather than be competing ideas or architectures. For this article we are focusing more on how they are similar or different rather than how they might work together (e.g., build an ADM on top of a data lake).
Below we have outlined the advantages and disadvantages of both approaches to help you make an informed decision.
ADM – advantages and disadvantages
An analytical data mart is a subset of a data warehouse that is designed for a specific business function or line of business. It contains a pre-defined set of data that is organized according to the needs of the business function. Analytical data marts are ideal for organizations that need to store and analyze structured data related to a specific function or process, such as finance, marketing, or sales. They offer fast query performance and easy data access for business users, making them an excellent choice for organizations with limited resources and specific data needs.
A structured ADM approach generally requires more initial work to connect to data sources, extract data from the source, restructure and prepare it, then store it in structured storage, such as a relational database (i.e., SQL Server, MySQL, etc.). This type of approach offers more control over the quality and consistency of data which is critical in data-driven decision-making processes. Data is organized into hierarchies and presented in multi-dimensional formats, enabling better query performance and improved end-user access. This option is ideal for organizations with a mature data warehousing strategy and ongoing needs for complex analytics.
As the size and complexity of data grows, it can be challenging to scale the ADM to meet the changing needs of the organization. Adding new data sources or changing the structure of the data requires significant resources and time. In addition, as the number of users accessing the data mart increases, query performance can be impacted, leading to slower response times.
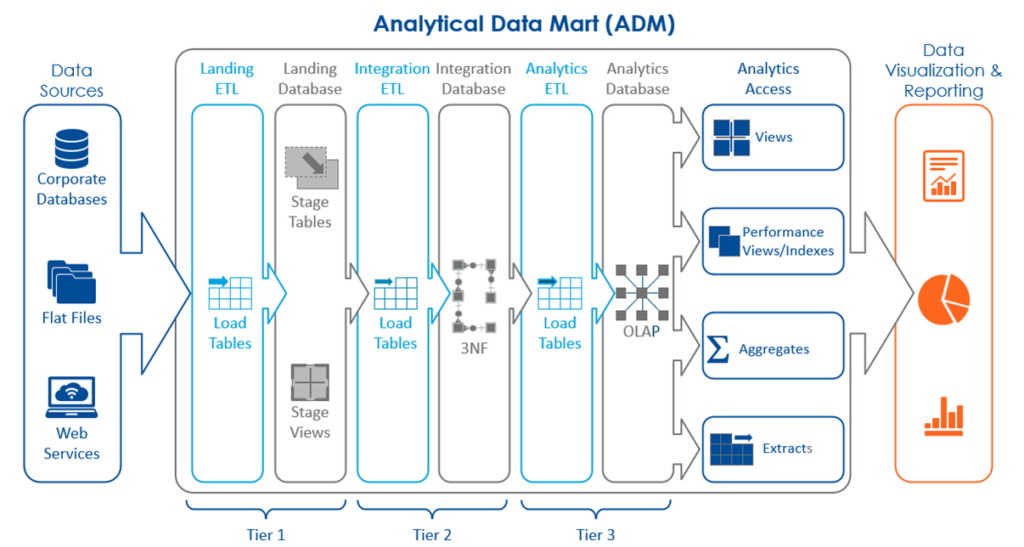
Analytical Data Mart (ADM) | |
Advantages | Disadvantages |
Fast query performance |
More work upfront |
More control over data quality and consistency |
Limited scalability |
Easy data accessibility for business users |
Potential data silos |
Cost-effective for specific data needs |
Can be complex to implement and maintain |
Ideal for complex analytics projects |
|
Data Lake – advantages and disadvantages
On the other hand, a data lake is more accommodating of unstructured data and stores it in raw form making the data lake approach a more flexible and cost-effective option. The data lake allows organizations to store data from multiple sources in their native formats without upfront transformation or restructuring requirements. Typically, data lakes are implemented using technologies/platforms like Hadoop or other big data technologies, providing an open but secure platform for storing and managing large volumes of data. Data lakes enable the easy addition of new data sources and support agile analytics through their flexible schema-on-read design approach.
However, data quality and consistency can become issues, as data is ingested from diverse sources without immediate consideration of its contribution to broader business objectives. In a data lake, data quality is the responsibility of the reader of the data. As a result, data quality management for a data lake is decentralized versus an ADM where it’s more centralized. With a data lake you need a higher number of people who know how to make sure data is clean and ready for use. Whereas, with an ADM only a few people must know how to ensure data quality. So more organizational “trust” of a broader set of people is required in a data lake environment which may make data quality control more onerous.
If adequate data quality and data governance measures are not implemented, a data lake will eventually degenerate into a data swamp. Data in a data swamp is either inaccessible to intended users or difficult to manipulate, and—inevitably—analyze.
Assessing the quality of data is always a challenge, and this challenge is amplified when working with large volumes of data stored in a data lake. One of the common mistakes organizations make is using a “schema on read” approach to access data without understanding the context in which the data was generated. This can result in drawing erroneous conclusions and making incorrect decisions based on flawed data. For example, if an e-commerce company is analyzing sales data without considering seasonality or market trends, they could make poor decisions about inventory management or pricing.
To avoid these issues, it is important to understand the context in which data was generated and ensure that data is properly structured before analysis. Implementing data governance policies and practices can help to ensure the credibility of the data obtained from a data lake. By taking these steps, organizations can more confidently analyze the data in their data lakes and extract valuable insights that can drive business success.
Data Lake | |
Advantages | Disadvantages |
Unlimited scalability |
Complexity of implementation |
Flexibility to store diverse data types |
Requires skilled resources for management |
Ability to handle large data volumes |
Potential data governance and security concerns |
Ideal for complex analytics projects |
Data quality and consistency may be challenging |
Ensure you choose the right approach
Choosing the right approach for architecting an analytics-supporting data repository is an important decision that can impact your organization’s ability to leverage insights from its data effectively. While both the ADM and data lake approaches have their own advantages and disadvantages, the choice ultimately depends on your organization’s specific needs and priorities.
Tools like Alteryx Server/Designer, Talend Open Studio, KNIME Analytics Platform, or Azure Data Factory can help to automate the process of cleaning, transforming, and validating data before analysis, ensuring that data is of high quality and can be trusted. However, different data preparation tools connect ADMs versus data lakes. Some tools also connect to both, so knowing which tools work for a particular data storage solution may take some investigation. A careful analysis of the benefits, limitations, and potential impacts on cost, scalability, and performance is essential before committing to either approach.
ADM approaches are ideal for enterprises with substantial structured data sources and complex queries where data quality and consistency are crucial. Data lakes are great for organizations that deal with diverse data from different sources and can benefit from agile analytics using a more flexible schema. In any case, it is necessary to remember that the implementation of a data repository to support your organization’s specific analytics requirements is a long-term investment, and it needs to be set up, managed, and maintained well to provide value over time.
A final thought
The reality is that data projects are never “done”, and the design of the analytical repository must support the future onboarding of new data assets or support the development of new analytical capabilities. Without the ability to grow and evolve, an analytical repository becomes less useful over time and eventually fades until it only delivers legacy reports that can’t be migrated to new platforms. One fundamental design premise for all data work that Optimus SBR delivers is extensibility. We design all analytical repositories with the premise that “things change” and new data or capabilities must be added. The architecture and the technology stack are designed to grow with the organization’s needs.
Optimus SBR’s Data Practice
We provide data advisory services customized to support the needs of public and private sector organizations. We offer an end-to-end solution, from data strategy and governance to data infrastructure, engineering, analytics, data science, visualization, insights, and training.
Contact Us to learn more about our Data practice and how we can help you on your data journey.
Industry Insights
Service Insights
Case Studies
Company News